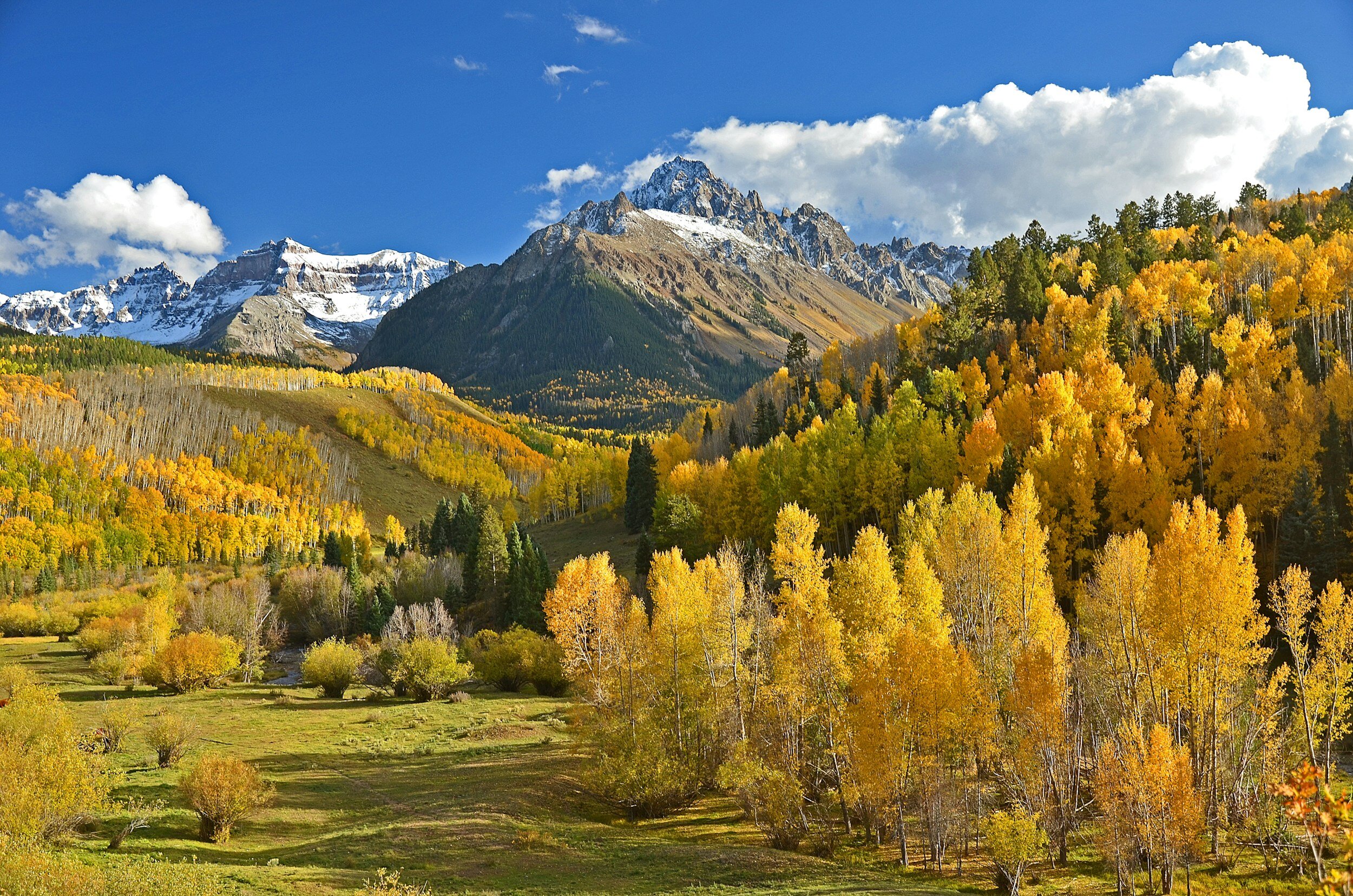
About me.
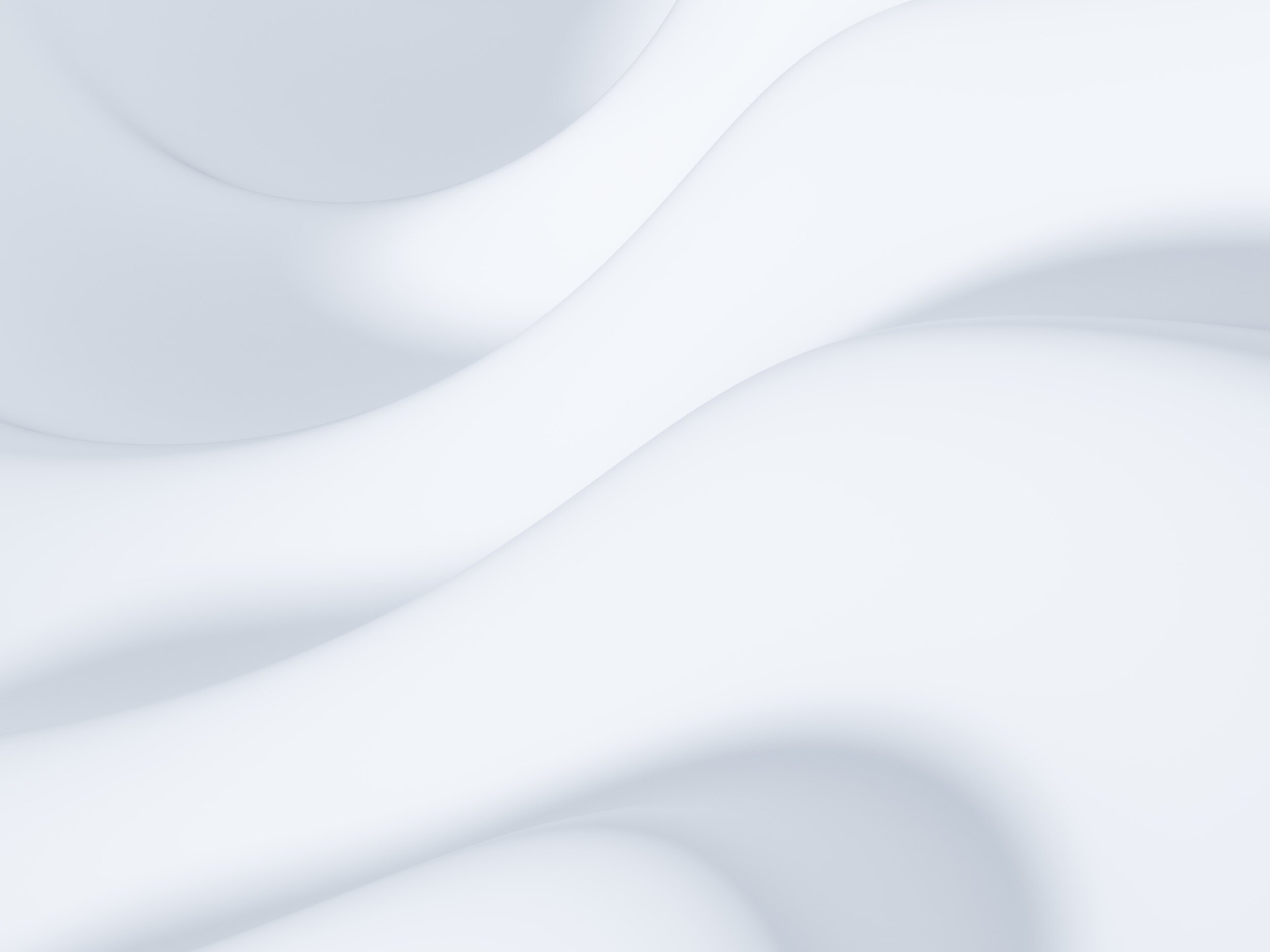
About Me
Hi! I'm Xavier, a published mathematician with a background in classical music, now focused on machine learning and time series analysis. My work focuses on modeling complex temporal patterns across domains—from physiological signals in healthcare to market dynamics in finance. I've worked in several research labs applying these methods to medicine, while actively developing my expertise in quantitative finance through projects and as a CFA Level I Candidate.
If you’d like a quick peak of what I’m working on, check the following topics below; otherwise more info about my background can be found at the bottom of the page.
-
Data in the real world is distributed through time. Time series modelling isn’t a new idea, dating back to the era of Galton (1880s), but still remains a significant challenge in deep learning. I enjoy working on unique and impactful problems within time series, where traditional deep learning paradigms fail. For example, many time series within healthcare (e.g., ECG, blood glucose measurements, sleep studies) are variable in length, yet, most of the current literature focuses on finite-context methods, which can only process sequences of fixed length. This need for robust variable-length time series classification (VSTC), is present not only in healthcare, but in many other real-world scenarios where there is no predetermined sequence length (e.g., finance, weather). We recently released a paper addressing this, called Stochastic Sparse Sampling: A Framework for Variable-Length Medical Time Series Classification—for more info on this, see my projects page.
-
While traditional deep learning methods provide point estimates—such as a single value or vector—they lack measures of uncertainty, limiting their usefulness in high-risk domains such as healthcare or finance. For example, incorporating uncertainty measures can be highly advantageous in clinical contexts, as it not only enhances patient outcomes but also improves clinician trust and promotes the adoption of models in the clinic. Currently, I am focusing on developing conformal prediction (CP) methods for time series forecasting of electromagnetic radiation to reduce radiation exposure. Conformal prediction is advantageous over other uncertainty quantification methods, as it provides a guarantee that prediction intervals contain the true outcome at a specified confidence level, regardless of data distribution or base model choice.
-
Currently I'm working on OpTrade, a framework for high-frequency forecasting of alpha term structures in American options markets using specialized deep learning architectures. My project aims to analyze market microstructure dynamics across various options contracts and eventually translate these insights into actionable trading signals. I'm particularly excited about my approach to position sizing based on model uncertainty using conformal prediction, which views alpha through the lens of uncertainty rather than traditional excess returns. This perspective allows me to capture more complex risk scenarios that standard volatility metrics might miss, potentially enhancing trading strategy performance in these sophisticated markets.
-
The rapid rise of AI in healthcare promises significant advancements, but there's a growing need for methods specifically designed to address clinicians' practical challenges and workflow. Currently, I am working on time series classification in seizure onset zone (SOZ) localization—the task of identifying seizure-inducing regions within the brain for epilepsy patients. Traditionally, neurosurgeons and epileptologists visually inspect intracranial electroencephalography (iEEG) signals to determine the SOZ. However, this challenge isn't so easy to apply any off-the-shelf machine learning algorithm. The signals can be extremely long (due to high sampling rate), variable in their length (for different recordings or patients), and the number of channels can vary among different iEEG setups. Furthermore, there are currently no clinically validated markers of the SOZ, and therefore there is a need of understanding the link between signal characteristics and pathology.
We just released a preliminary version of our work surrounding this at the NeurIPS 2024 Workshop on Time Series in the Age of Large Models, with the full version on arXiv and under review. Our approach, called Stochastic Sparse Sampling (SSS), can handle not only variable-length and long sequences, a varying number of channels, but also provides post-hoc model interpretability with respect to local signal regions, which are directly tied to the probability of the SOZ. For more information on this, see my projects page.
My background
Currently, I am a MSc in Computer Science student at the Lassonde School of Engineering, York University within the NWGNR Lab. My thesis focuses on the development of novel deep learning methods for time series analysis, with an emphasis on uncertainty quantification, and how to adapt current methods to irregular time series (e.g., variable-length, irregularly-sampled). I work in parallel with the NSBPSL within the Krembil Research Institute at Toronto Western Hospital with a focus on developing state-of-the-art methods for interpretation of neurological diseases in time series data, and their clinical applications. I was also a research intern at T-CAIREM, where I advanced SSL techniques with GNN encoders to boost seizure detection downstream, for which I am continued my work on seizure onset zone (SOZ) localization that was accepted to the NeurIPS 2024 Workshop on Time Series in the Age of Large Models. During my undergrad I had the privilege of holding the NSERC USRA position twice at York University where I worked on algebraic combinatorics and operator algebras. I also have a fascination with quantum computing, which led me to participate in the esteemed USEQIP program at the Institute for Quantum Computing, University of Waterloo. Before all of this, my research journey began at the Population Neuroscience & Developmental Neuroimaging Lab within Holland Bloorview Kids Rehabilitation Hospital.
-
MSc in Computer Science — York University (2023—2025)
Specialized Honours BSc in Mathematics — York University (2019—2023)
Music Industry Arts — Fanshawe College (2017—2019)
Schulich School of Music — McGill University (2013—2015)